Smart Maintenance in the railway industry means to leverage the potential of digitalization. Possible solutions include the optimization of existing maintenance processes, reducing life cycle costs by efficient use of existing assets and implementing new business models and services. Sense and connect is an important topic, availability and utilization of data from different sources are deciding factors for success. Infrastructure to create and handle a large amount of data is needed. Technologies for data analytics, knowledge discovery and artificial intelligence developed rapidly in the last years and are core competencies for the next decade. Deployment and application of new knowledge is just as important and a great challenge when implementing Smart Maintenance.
What is Smart Maintenance and why do we need it?
Data analytics creates models out of data with the use of machine learning and modern statistical methods. To provide the right data a working data management is crucial. This includes the data architecture, data storage and data transfer. General questions like “edge vs. cloud architectures” or “stream vs. batch processing” must be discussed and answered for the individual use case.
Before any data can be analyzed the data needs to be recorded. Onboard and wayside sensors are getting more common. Data exchange between rail operators and infrastructure operators can be leveraged for success. Additional available data is getting more frequent, good examples are drone footage or satellite data for health assessment of the infrastructure. Moreover, manual data like damage reports are easily available in modern systems. Enhancing factors are that trust in cloud systems is increasing, computing power and storage is getting cheaper and machine learning is improving rapidly.
With the correct data and a working data pipeline to analyse data, a health assessment can be implemented. The most common approaches are Condition Based Monitoring to evaluate the current health status of a component and Predictive Maintenance to predict the remaining useful life. The next steps will be Prescriptive Maintenance, which automatically generates advice on operating conditions and intelligently schedule and plan maintenance.
The process follows up with the use of the generated knowledge about the system health for maintenance planning to generate economic value. Further systems like enterprise resource planning (ERP), incident management system (IMS), job scheduling and part management systems can be integrated in a holistic solution.
The last step is to integrate the previous results in the maintenance execution. Condition diagnosis currently takes up 30% of the work in maintenance execution. Studies show that these costs can be reduced by 18% with the use of Smart Maintenance. Intelligent systems which offer new solutions for workers can raise efficiency even more. An overview of a Smart Maintenance system can be found in Figure 1.
How does Smart Maintenance work?
Traditionally maintenance in the railway sector is done on a strict schedule based on calendar or run time, as well as repairs when a component fails. This means that maintenance work is currently executed either to early or too late for the requirements of a specific component. As more data gets available the concept will change from reactive to Predictive Maintenance as described before. It means that a health assessment can be done virtually and continuously.
There are different development stages of the health assessments. Condition Based Maintenance can evaluate the current heath (status) of a component. The simplest implementation would be a categorization in two states: working and not working. Further development could add more states, for example: good as new, working well, first signs of damage, not working. As models evolve towards Predictive Maintenance, the challenge is to predict how far the state not working is in the future. This value is usually described as remaining useful life. In most cases a model is trained with machine learning. After testing the model, it can be applied to the live data to predict the remaining useful life. This value is then used to schedule maintenance in time or save costs when components are available longer than expected.
How can you implement Smart Maintenance?
More systems get digitalized every year and most companies have some data available by now. The type of data may vary from case-to-case, but the data is rarely used for further analytics and to implement Condition Based or Predictive Maintenance. To address this topic, it is important that a company has a data strategy to bundle efforts and be cost effective. Smart Maintenance has huge potential, but the choice of the correct use case should be taken with care before spending time and effort. Additionally, a structured approach is needed to be successful with multiple use cases.
First, an initialization phase should be done, for which an interdisciplinary team of technology and domain experts is required. It is important to include all stakeholder groups in the process. Domain experts can give valuable insights to business details and can validate use cases. Technology experts can give their judgement what is possible with the current infrastructure and what is missing for future projects. After defining one or multiple use cases a first quick check can be done. Data availability, quality and quantity should be analysed, and an explorative data analysis can be conducted. First hypothesis can be tested, anomalies and patterns can be found.
If the first phase is successful, the next step is developing a working system. Software development processes are important for success. A professional development team will handle architecture, integration (CI/CD), validation, review and testing, documentation. Besides all the classical software development topics the data analytics challenges like feature engineering, choosing frameworks and algorithms, model training and optimization and visualization need to be handled.
After finalizing a working analytic system, the results must be integrated to operation. Maintenance execution systems and tool chains vary, and interfaces between solutions need to be adapted accordingly. After operationalizing the analytic system continuous validation is key to long term success. Parameters of the system can change e.g., the infrastructure setup might change over time. Performance should be monitored to detect model drifts and adjustments can take place accordingly. An elegant solution would be systems which continue to learn while operation is ongoing. Certification of such systems is, especially in Europe, an ongoing challenge but processes are expected to change as well.
Conclusion
Smart Maintenance is one of the core innovative topics for rail and a direct result of the ongoing digitalization in the railway industry. Costs can be reduced, and availability of rolling stock and infrastructure can be increased significantly. Maintenance systems are developing from reactive behaviour after component failure or strict time-based cycles towards proactive maintenance. Railway systems vary and Smart Maintenance can be addressed in different ways, but first results can be achieved fast, without a huge amount of effort. A structured approach can help to save resources and avoid mistakes, which have a long-term business impact. As this is an innovative topic there are still challenges, and new ones will occur as new systems get implemented for more use cases. ITK Engineering is open to collaborate in Smart Maintenance with partners from the railway industry.
Websites
We are not responsible for the content of external sites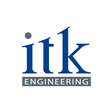